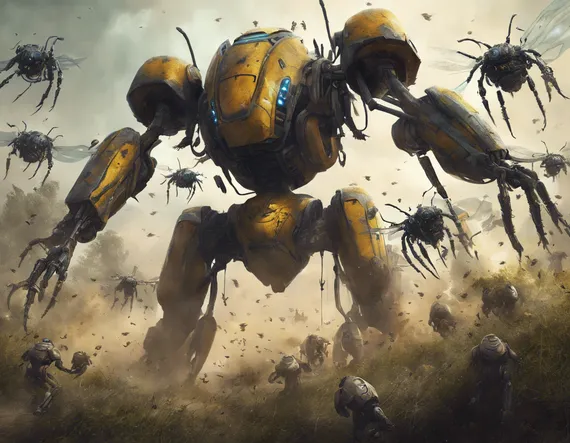
OpenAI's Swarm vs. AutoGen: A Quick Comparison
Swarm's simplicity is the point, but AutoGen's flexibility is the draw.
13 Oct, 2024 - 04 Mins read
This Easter weekend, I forbade myself from working. I half-succeeded: although I made two AutoGen projects, none were for my day job! #soproud Here goes: a cover letter generator and a multi-provider therapy session. (A friend needed both and I thought it would be a good distraction for us to convert a human problem into a technical problem. Because that’s healthy.)
But first, what is AutoGen?
AutoGen lets you write agents, which is a fancy word for “LLM prompts with tool access and execution environment”. Furthermore, it lets you compose said agents into multi-agent workflows, which allow the agents to respond to each other based on the conversation history and each agent’s prompt.
This is useful to you if you often go back-and-forth with ChatGPT to iterate towards a final result in a way that could be taught to a set of interns. This is basically a way to let the machine take your turn in the conversation and “steer the ship” towards a goal you’ve set out for it.
AutoGen does this programmatically in Python, but the maintainers also released a GUI named “Autogen Studio”, which is a little more user-friendly. The remainder of this article will focus on the Python side of things, though.
The concept behind each project was simple:
pyautogen
.UserProxyAgent
as a stand-in for the user. While by default, the UserProxyAgent
prompts for human input every time it’s invoked, it does not need to, and you can use it just to simulate the opening of the conversation.GroupChat
.(I should really make a Copier template for this. Of course, it’s a little complicated by the fact that the nature, prompt and setup of each agent is a little different each time, but there’s sufficient similarity that it might be worth it.)
I want to talk about the cool parts of the process.
I think this is the most important part of the process to get right, especially when making a multi-agent process for a highly personalized activity like therapy or job applications. Of course, you should follow the best practices for prompt engineering, but if this is a task you already have some experience in, you should strive to make the secret sauce explicit.
To take the example of the cover letter writing:
Or, of course - I know the kind of therapy I like, and my friend knew what kind of therapy he prefers. So we could made the prompts specific to our needs. This is a challenge in putting the prompts out there, in fact - both the cover-letter and the therapy session requirements can get a little too personal.
This is the trickiest part to me, as the definition of finite-state machine transitions is the same for both success and failure - which means it needs to permit for both, confusing either.
This was probably the toughest. Two reasons here: (1) developing evals themselves is difficult, and (2) the cost of running the evals is high, so you don’t want to run them too often, which means you don’t get as much feedback as you’d like as often as you’d need.
(This is where Small Language Models could shine, in theory! But then you have to adapt the prompts to different models, and that’s a whole other can of worms.)
This doesn’t have a good answer yet, even though it’s a problem that’s been solved many times over. The Teachability
feature is a sort-of too-smart solution to a different problem, which is that agents don’t remember dynamically what you told them - but what you often want is a fully detereministic key-value store. Well, guess what - fully deterministic key-value stores are not exactly uncommon in the tech space! But Autogen doesn’t support any of them out-of-the-box, so there’s a wide space of custom implementations.
By default, there isn’t much - on a run that completes gracefully, you get an object with .chat_history
, otherwise you get a traceback. There’s some default instrumentation, but none that appears easily extensible.
Each run cost between $0.05 and $0.50, depending on the length of the conversation, using gpt-4-turbo-preview
. This is a little expensive - I still wouldn’t hesitate to use it for a personal use case, but would likely balk at providing it as a free service to the general public. (Unless it’s “bring your own API key”, I suppose.)
Multi-agent workflows impress me. In specific use cases, they’re a clear step above bare GPT-4 prompting - and even though they’re not a panacea, the list of shortcomings is highly tractable. I’m looking forward to shortening it.
Swarm's simplicity is the point, but AutoGen's flexibility is the draw.
13 Oct, 2024 - 04 Mins read
Quick practitioner's notes on actual usage.
20 Jul, 2024 - 03 Mins read
If you're looking for an data-driven AI consultant or simply want to have a chat.