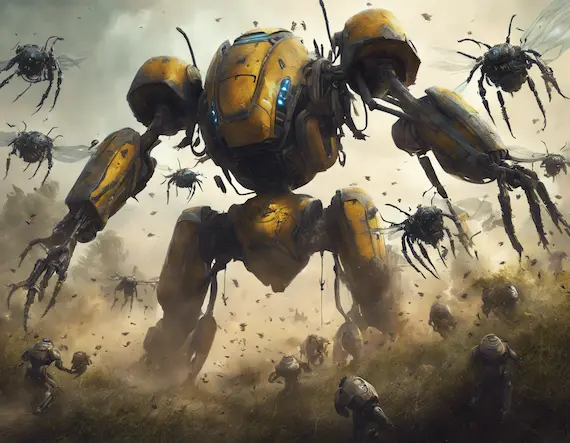
OpenAI's Swarm vs. AutoGen: A Quick Comparison
Swarm's simplicity is the point, but AutoGen's flexibility is the draw.
13 Oct, 2024 - 04 Mins read
A recent LLM project required a locally-hosted solution for tracing and prompt management. I’ve been eyeing Langfuse, which integrates low-level tracing (á la LangSmith, Logfire or LangTail) with prompt management and a usage dashboard (as well as other features) - but you can deploy it on your own infrastructure without paying up the wazoo.
Two straightforward usage issues came up in the process: how to patch OpenAI Chat Completions endpoint with multiple OpenAI-patching libraries, and how to set up a prompt management workflow. Since I couldn’t find any write-up online or related messages on the many associated Discord servers, I’m noting my solution here.
A lot of libraries accomplish their functionality by plugging into the official OpenAI Python SDK. If you want observability and functionality, you have to combine them. How?
Well, one at a time, and you hope they don’t do anything to break compatibility in the future.
from instructor import AsyncInstructor, from_openai
from langfuse.openai import AsyncOpenAI
def get_instructor_client(api_key: str | None = None) -> AsyncInstructor:
"""Get an OpenAI-compatible client."""
raw_client = AsyncOpenAI(api_key=api_key)
client = from_openai(raw_client)
return client # type: ignore # noqa: PGH003
The last line gives mypy
the heebie-jeebies for no clear reason - if the initial import is from openai import AsyncOpenAI
, everything is typed as intended, and langfuse.openai.AsyncOpenAI
doesn’t seem to be typed differently - but the solution works like a charm.
(I don’t want to think too hard about throwing LiteLLM, OpenRouter or RouteLLM into the mix, though.)
If setting up Langfuse isn’t the first thing you’ve done in your LLM project, you probably have existing API calls using hard-coded prompts. At this point, you face the choice: do I manually transfer the prompt to the Langfuse UI, or do I run a single-purpose script that sets up the prompts in Langfuse? And if the latter, at what point in the lifecycle of the app do I re-run this script? And how do I make sure this doesn’t create new prompt versions in vain, since Langfuse prompt creation is not yet idempotent?
The solution I came to uses a Python workflow: assume the prompt exists in Langflow and if not, create its first version. Afterwards, continue iterating on the prompt in the Langflow UI.
The following excerpt loads - and, upon failure, defines - a chat-extract-feedback
chat prompt. After the load/create step, everything proceeds normally.
(In this case, this is part of a fictional application named Weathervane, which defines Pydantic models of input and output in weathervane.models
. See here for an introduction to Pydantic/Instructor.)
from instructor import AsyncInstructor
from langfuse import Langfuse
from langfuse.api.resources.commons.errors.not_found_error import NotFoundError
from weathervane.models.chat import IncomingChat
from weathervane.models.output import ExtractedFeedback
async def llm_extract_feedback_from_chat(client: AsyncInstructor, chat: IncomingChat) -> ExtractedFeedback:
"""Extract feedback from a chat message via LLM call."""
langfuse = Langfuse()
try:
prompt = langfuse.get_prompt("chat-extract-feedback", type="chat", label="production")
except NotFoundError:
prompt = langfuse.create_prompt(
name="chat-extract-feedback",
prompt=[
{"role": "system", "content": "Extract feedback information from the chat message."},
{"role": "user", "content": "{{chat_json}}"},
],
config={
"model": "gpt-4o",
# "response_model": ExtractedFeedback,
},
type="chat",
labels=["production"],
)
compiled_prompt = prompt.compile(chat_json=chat.model_dump_json())
return await client.chat.completions.create(
response_model=ExtractedFeedback, # kwarg added by Instructor
messages=compiled_prompt,
langfuse_prompt=prompt, # keeps the version in Generations
**prompt.config,
)
(The only exception to the normality of this is that Langfuse currently cannot version Instructor/Pydantic models passed in response_model
, or at least their schemas. This is unfortunate, since the response schema is an essential part of the prompt, but can be worked around.)
@observe
and FastAPIThis works:
@router.post("/chat")
@observe()
async def extract_feedback_from_chat(
email: IncomingChat, client: Annotated[AsyncInstructor, Depends(get_instructor_client)]
) -> ExtractedFeedback:
"""Extract feedback from a chat."""
# Group the following traces by session
langfuse_context.update_current_observation(session_id=str(uuid4()))
This doesn’t:
@observe()
@router.post("/chat")
async def extract_payment_info_from_chat(...) -> ...:
Neither order breaks FastAPI, but the latter breaks Langfuse.
I’m still finding my way around some of Langfuse’s more advanced features, and figuring out whether they can form the foundations of an AI engineering flywheel - the sort that Shreya Shankar writes about. I do think it’s a good observability platform that will allow for a measure of debugging and explainability of some of the more complex prompt chains, and possibly some amount of evaluation. That’s a good start.
Swarm's simplicity is the point, but AutoGen's flexibility is the draw.
13 Oct, 2024 - 04 Mins read
A reflection on the process of creating multi-agent workflows with Autogen.
15 Apr, 2024 - 05 Mins read
If you're looking for a skilled data engineer passionate about transforming data into actionable insights or simply want to have a chat.